Role of Artificial Intelligence (AI) in Neuromuscular & Electrodiagnostic Medicine
The relationship between AI, and the sub-components of machine learning (ML), and deep learning (DL) are illustrated in the figure below, and the topic is further elaborated in the article “The role of artificial intelligence in electrodiagnostic and neuromuscular medicine: Current state and future directions”, published in December 2023 in Muscle & Nerve. 17
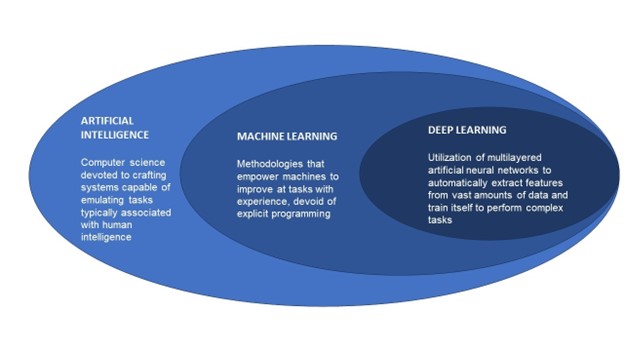
Below is a glossary of other common terms used when discussing AI:
TERM | DEFINITION |
Supervised Learning | Machine learning that is based on input–output pairs. It uses labeled datasets to train algorithms to classify data or predict outcomes accurately |
Unsupervised Learning | Machine learning algorithms which analyze and cluster unlabeled datasets, thereby disclosing hidden patterns or data categorizations without the need for human intervention |
Artificial Neural Network (ANN) | Computing process that analyzes data by mimicking synaptic connections |
Deep Neural Network (DNN) | Brain-like multilayer artificial neural network which further enhances complex data analysis and deep learning |
Natural or “Large” Language Model (LLM) | A deep learning algorithm which can recognize, summarize, translate, predict, and generate language content using very large datasets |
Generative Pre-trained Transformers (GPT) | These are a family of neural network models that uses the transformer architecture, giving applications the ability to create human-like text and content, and answer questions in a conversational manner |
Chatbot | Computer program that simulates conversation with a human |
AI’s foray into medicine can be traced back to a patient interactive system, ELIZA, which was an early natural language processing computer program developed at MIT in the mid-1960s, but further development was limited by available computing power 3. There have been significant strides over the past decade, and much more advanced systems have emerged, including GPT-4 which was introduced in 2023. That system can accurately answer most questions on the medical licensing exam, correctly answer most questions from patients or physicians, and could have done the initial draft of this paper. The system “self-trains” by accessing data available on the internet, online textbooks, websites, podcasts etc. Other systems being developed and becoming available have the potential to “listen” to the conversation between a patient and provider and produce a draft of the clinical note4, and even possibly cue up orders for providers to review before signing.
Physician/Provider Aspects
As much as the application of AI to healthcare is exciting and promising, providers must exercise caution with full awareness of the limitations and risks involved. Among such considerations is the responsible development of rigorously validated AI technologies which requires that models are trained adequately, transparently and with sufficiently diverse populations to reflect those to whom the technologies will be applied. This is necessary to mitigate the potential disadvantages and harms to underrepresented groups, including minorities. With the rapid pace of development in the field of AI in general, and in healthcare in particular, regulatory measures are significantly lagging, and it is imperative that this limitation is curtailed in the interest of patient safety. Related are novel challenges with ethical and legal issues pertinent to patient privacy (including cybersecurity), and liability for medical errors in which there may have been AI over-reliance. Accordingly, it is important that AI should serve as a clinical decision support tool rather than a replacement for human expert judgement. Providers should be adequately trained in the clinical application of AI technologies and continuously monitor the performance of these to ensure optimal efficacy and risk minimization.5
Patient/Public Aspects
AI and patient care: AI can assist in gathering medical data to assist in accurate and timely diagnoses. AI can recognize patterns in NM medicine and identify early signs of diseases that might be overlooked by human physicians It can also help physicians implement early preventative measures.to avoid complications, thereby leading to better health outcomes. 1,2
AI and patient management using evidence-based medicine: AI can assist in identifying and providing evidence-based treatment recommendations considering unique patient related factors. It can support clinical decision-making in conjunction with human judgment and thus help optimize efficacy and patient safety. 1
AI role in administrative processes and patient experience: AI can facilitate patient scheduling, as well as assist with coding, billing, prior authorizations and appeal letters for insurance coverage denials. This can lead to improved patient-provider interactions including “liberated” time that could be used for more face-to-face time during encounters, thereby promoting overall better patient experiences.
AI and ethical considerations in patients’ privacy and safety: Prior to implementing AI in patient care, careful consideration must be given and strict regulations enacted to protect patients’ privacy and ensure data security. Furthermore, the same caution which would be prudent for any new medical technology must be exercised and rigorous oversight will be needed prior to and throughout implementation of any AI programs in healthcare. Some major areas of concern specific to AI in healthcare include:
Informed Consent: AI programs may have access to or utilize patient information that the patient has not actually consented to releasing. Care must be taken to understand what information is being used and how it is being used so that patients can be properly informed in an easy-to-understand format and, thus, be able to provide appropriate consent. 3
Bias and discrimination: AI may base consideration of information and/or recommendations on well-known (or yet-to-be discovered) biases that currently exist in available data sets used for the AI model training. 3
Human autonomy: There must be human oversight of any AI programs used in patient care to ensure that human values and ethical considerations are protected and included in any AI-assisted medical decision-making. 2
The World Health Organization (WHO) has identified 6 core principles to consider when utilizing AI in healthcare10:
Protect autonomy;
Promote human well-being, human safety, and the public interest;
Ensure transparency, explainability, and intelligibility;
Foster responsibility and accountability;
Ensure inclusiveness and equity; and
Promote AI that is responsive and sustainable.
Working with Industry, and Regulatory Aspects of AI in EDX and NM Medicine
As previously alluded to, with the very rapid development in this complex technical area, regulatory bodies have struggled to keep up and provide the necessary guardrails for AI applications in medicine.
The US Food and Drug Administration (FDA), for instance, has imposed rigorous requirements for medical devices and algorithm licensing. 11,12 In response to the evolving nature of technologies, the FDA has introduced a new regulatory strategy called the Total Product Lifecycle (TPLC), which allows for continuous modifications of algorithms as they develop and learning occurs over time, enabling effective regulation of Software as Medical Devices (SaMD) throughout their lifecycle.
The FDA's regulatory process for algorithms comprises three licensing pathways: the Pre-Market Approval pathway, involving a comprehensive review of the algorithm's safety and efficacy before clinical use; the 510(k) pathway, which involves a comparison study with other similar devices/algorithms; and finally, the De Novo pathway, where safety and efficacy are studied for algorithms without comparable devices. 11 To date, the FDA approved over 500 AI/ML-enabled medical devices, with some of these devices utilizing electromyography signals to aid prosthetic movements. However, the focus of most approved algorithms are the fields of radiology and cardiology applications, with only few examples notable for neurology and NM medicine, in particular.
Despite the relatively slow FDA approval process, there remains a significant lack of a robust framework for assessing and regulating algorithms applied in the medical field, particularly in neurology. A recent systematic review of 53 algorithms with applications in the central nervous system revealed a concerning lack of substantial peer review, with only 10 algorithms being published, and a scarcity of rigorous clinical efficacy studies, often involving biased study populations. 13 These findings underscore the importance of advocating for and actively participating in the creation of regulatory frameworks for the use of AI/ML in neurology and NM medicine. This is particularly important in preventing unforeseen and unintentional harms which may arise from the premature deployment of healthcare AI applications which may have had suboptimal development (including inadequate AI model training) and pre-market testing.
The implementation of practical AI use from the research setting to the clinical setting is complex. There are many potential areas that need crucial oversight. Judicious regulation must be employed to prevent harmful outcomes and the loss of public trust. It is imperative for AI technology applications to be safe, accurate and efficacious. For this, it is necessary to use specific quantitative metrics to validate the technology. However, it must be noted that these metrics cannot always account for nuances that occur in specific fields. 14 Combining the knowledge and experience of health care professionals with these quantitative metrics is essential to improving its real-world performance. AI technologies should not be regarded as a replacement, but rather an enhancement for clinical decision-making. Frequent reassessment of the technology’s performance must be implemented to ensure continued safety and reliability.
Since AI/machine learning is heavily based on data input, there must be transparency regarding the data provided to training algorithms, and this data must be properly assessed for bias. It is known that if given biased data, AI can reproduce and amplify patterns of preexisting inequality and discrimination, and this must be proactively prevented. 15 Additionally, it is critical to safeguard patient privacy and autonomy. Accordingly, there should be adequate disclosure of AI usage if applied to a service or product received in healthcare. Another important consideration is AI technology’s effect on healthcare workers. Electronic health records were touted to improve efficiency and quality of work, but it has contributed significantly to increased healthcare worker burnout. 16 Accordingly, careful consideration is needed when integrating AI into clinical workflows.
In summary, there is great potential for improvement of clinical work and patient care with the use of AI in neurological and NM medicine. An effective regulatory approach should balance innovation with patient safety, data privacy, equity, and clinical efficacy. Collaboration and cooperation between professional societies, government, and industry is needed to ensure successful integration of this powerful technology into clinical use.
References
Haenlein M, Kaplan A. A Brief History of Artificial Intelligence: On the Past, Present, and Future of Artificial Intelligence. https://doi.org/101177/0008125619864925 [Internet]. 2019 Jul 17 [cited 2023 Oct 21];61(4):5–14. Available from: https://journals.sagepub.com/doi/abs/10.1177/0008125619864925
Augmented intelligence in medicine | American Medical Association [Internet]. [cited 2023 Oct 21]. Available from: https://www.ama-assn.org/practice-management/digital/augmented-intelligence-medicine
Weizenbaum J. ELIZAa computer program for the study of natural language communication between man and machine. Commun ACM [Internet]. 1966 Jan 1 [cited 2023 Oct 21];9(1):36–45. Available from: https://dl.acm.org/doi/10.1145/365153.365168
Automated AI medical scribe helps doctors avoid burnout | AI Magazine [Internet]. [cited 2023 Oct 21]. Available from: https://aimagazine.com/articles/automated-ai-medical-scribe-helps-doctors-avoid-burnout
Khan B, Fatima H, Qureshi A, Kumar S, Hanan · Abdul, Hussain J, et al. Drawbacks of Artificial Intelligence and Their Potential Solutions in the Healthcare Sector Human immunodeficiency syndrome SARS Severe acute respiratory syndrome NHS National Health Service FDA Food and Drug Administration. Biomedical Materials & Devices [Internet]. [cited 2023 Oct 21];1:3. Available from: https://doi.org/10.1007/s44174-023-00063-2
Artificial Intelligence in Emergency Medicine: Benefits, Risks, and Recommendations. 11 Feb 2022 DOI: https://doi.org/10.1016/j.jemermed.2022.01.001
Implementing Artificial Intelligence in Health Care: Data and Algorithm Challenges and Policy Considerations. American Journal of Biomedical Science and Research. 8 Jan 2021. DOI: http://dx.doi.org/10.34297/AJBSR.2021.11.001645
The ethical use of Artificial Intelligence in Nursing Practice. (n.d.). https://www.nursingworld.org/~48f653/globalassets/practiceandpolicy/nursing-excellence/ana-position-statements/the-ethical-use-of-artificial-intelligence-in-nursing-practice_bod-approved-12_20_22.pdf
Khullar D, Casalino LP, Qian Y, Lu Y, Krumholz HM, Aneja S. Perspectives of Patients About Artificial Intelligence in Health Care. JAMA Netw Open. 2022;5(5):e2210309. doi:10.1001/jamanetworkopen.2022.10309
World Health Organization. (n.d.). Who calls for safe and ethical AI for health. World Health Organization. https://www.who.int/news/item/16-05-2023-who-calls-for-safe-and-ethical-ai-for-health
Food and Drug Administration, 2019. Proposed regulatory framework for modifications to artificial intelligence/machine learning (AI/ML)-based software as a medical device (SaMD).
Benjamens, S., Dhunnoo, P. and Meskó, B., 2020. The state of artificial intelligence-based FDA-approved medical devices and algorithms: an online database. NPJ digital medicine, 3(1), p.118.
Yearley, A.G., Goedmakers, C.M., Panahi, A., Rana, A., Doucette, J., Ranganathan, K. and Smith, T.R., 2023. FDA-approved machine learning algorithms in neuroradiology: A systematic review of the current evidence for approval. Artificial Intelligence in Medicine, p.102607.
Thomas RL ,Uminsky D. Reliance on metrics is a fundamental challenge for AI. Patterns (N Y). 2022; 3100476.
Leslie, D. (2019). Understanding artificial intelligence ethics and safety: A guide for the responsible design and implementation of AI systems in the public sector. The Alan Turing Institute. https://doi.org/10.5281/zenodo.3240529
Kevin B. Johnson, MD, MS1,2; William W. Stead, MD3. Making Electronic Health Records Both SAFER and SMARTER. JAMA. 2022;328(6):523-524. doi:10.1001/jama.2022.12243
Taha MA, Morren JA. The role of artificial intelligence in electrodiagnostic and neuromuscular medicine: Current state and future directions. Muscle Nerve. 2023 Dec 27
Document History
Creation of New Guidelines, Consensus Statements, or Position Papers
AANEM members are encouraged to submit ideas for papers that can improve the understanding of the field. The AANEM will review nominated topics on the basis of the following criteria:- Members’ needs
- Prevalence of condition
- Health impact of condition for the individual and others
- Socioeconomic impact
- Extent of practice variation
- Quality of available evidence
- External constraints on practice
- Urgency for evaluation of new practice technology